

#WIRESHARK SUM IOGRAPH VERIFICATION#
This article suggests limiting the response time for each request from the server, and in dealing with response, it can use a latest verification system.
#WIRESHARK SUM IOGRAPH HOW TO#
The critical analysis of the current literature provides insights on how to mitigate and prevent these types of attacks. The objective of this review paper is to provide the readers with the information on DoS attacks and the attackers’ intention behind these illegal activities. Several mitigation techniques are available but these techniques are still not sufficient to detect and combat the malicious requests. This prevents the server from providing services to the legitimate users which leads to organizations and businesses losing millions of dollars. It disallows the services of an organization to be offered to users by flooding the server with legitimate request thus exhausting the server. It is common because it is very easy to implement/setup. Hyper Text Transfer Protocol (HTTP) Get attack is the most common type of Denial of Service attacks (DOS) found today. On each DDoS attack, we evaluated the effectiveness of the classification methods Logistic regression, Decision tree, Random Forest, Ada boost, KNN, and Naive Bayes, and determined the best classification algorithms for detection. It contains eleven different DDoS attack datasets in CSV file format. We used the CICDDoS2019 dataset which is collected from the Canadian Institute of Cyber security in this study. This study used six machine learning classification algorithms to detect eleven different DDoS attacks on different DDoS attack datasets. But researchers, use individual classification algorithms on generalized DDoS attacks.
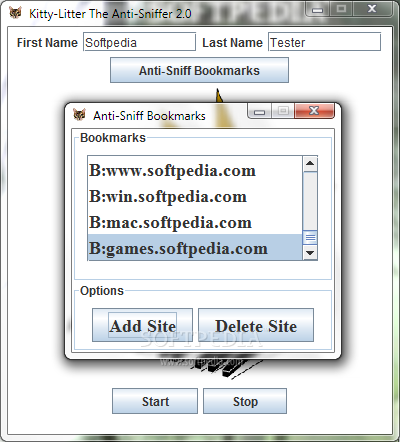
Researchers get good accuracy values while detecting DDoS attacks by using classification algorithms. In order to detect DDoS attacks, statistical and data mining methods have not been given good accuracy values. It shows the importance of effective DDoS detection mechanisms to reduce losses. It leads to so many negative consequences in terms of business, production, reputation, data theft, etc. A Distributed Denial of Service (DDoS) attack is one of the most common cyberattacks that has affected availability, which is one of the most important principles of information security. The simulation results prove the effectiveness of the proposed mechanism, compared with the existing systems.Ĭyber attacks are one of the world's most serious challenges nowadays. This paper introduces a novel mechanism based on a Bayesian model to detect abnormal data traffic and discriminate DDoS attacks from FC in it. The detection of such abnormal traffic and then separation of DDoS attacks from FC is also a focused challenge. Both DDOS and FC are considered abnormal traffic in communication networks. On the other hand, legitimate users may produce a larger amount of traffic known, as the flash crowd (FC). Most of the DDoS detection systems rely on the analysis of the flow of traffic, ultimately with a conclusion that high traffic may be due to the DDoS attack. The deployed environment of WSNs is noncentral, unattended, and administrativeless therefore, malicious attacks such as distributed denial of service (DDoS) attacks can easily be commenced by the attackers. The nodes in WSNs, due to their vulnerable nature, are always prone to various potential threats. These networks are deployed in such places where the repairments, in most cases, become difficult. Wireless sensor networks (WSNs) are low-cost, special-purpose networks introduced to resolve various daily life domestic, industrial, and strategic problems.
